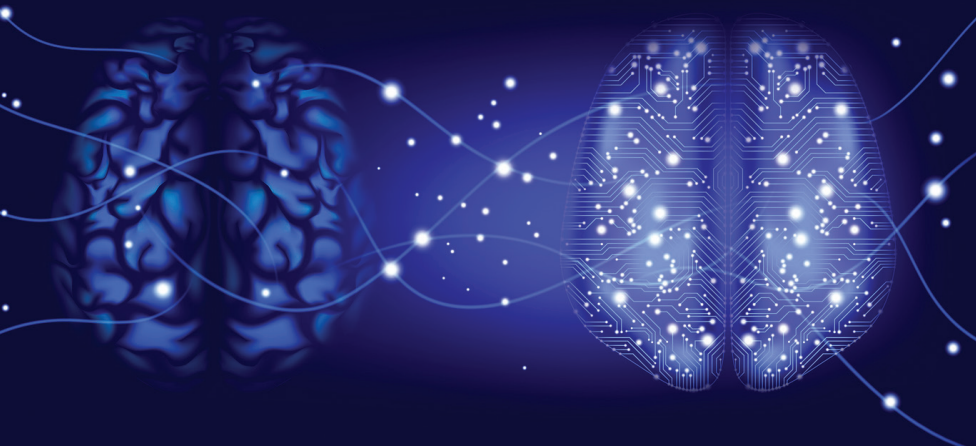
MicroStrategy, AWS Eye Challenges and Opportunities in AI, Machine Learning
There are opportunities still awaiting media and entertainment and other organisations who have yet to take full advantage of artificial intelligence (AI) and machine learning (ML) but challenges remain as well, according to Amazon Web Services (AWS), MicroStrategy and cloud computing-based data warehousing company Snowflake.
“We’ve seen a lot of use cases and trends at AWS” when it comes to AI and ML – a “broad spectrum, from domain-specific trends around enterprise AI” and taking ML workloads from experimentation to production and deploying that responsibly, Dylan Tong, global technical lead of AI augmented analytics at AWS, said Feb. 1 at MicroStrategy World 2002, during the panel session “Democratising AI & Machine Language Workflows.”
“We’ve seen trends and use cases specific to industries like healthcare, manufacturing [and] supply chain,” he said. There are also “broad megatrends like democratisation,” he noted.
“Specifically, in the analytics space, there’s trends around how we can empower non-data scientists, like the data analysts and data engineers, so that they can take a greater ownership of the machine learning development process [and] maybe build their own production-quality models and create their own kind of predictive solutions that deliver business foresight,” he explained.
“We all know implementing new products or adopting those new technologies can be complicated,” Alberto Abrisqueta, product manager at MicroStrategy and moderator of the online panel, pointed out.
Democratizing AI, ML
“What are the challenges organisations face when democratising AI and machine learning?” Abrisqueta asked.
Responding, Andries Engelbrecht, partner solution architect at Snowflake, said: “Many organisations are adopting a data-centric approach to machine learning, where they find that adding additional data, or relevant data, to the ML process produces better results as opposed to tuning and optimising these algorithms and models. The challenge with that, however, is that the organisation’s data may be in various silos by different departments or different functions or even various locations.”
From MicroStrategy’s perspective, “one of the big” challenges that organisations are facing throughout this process is trust, according to Amit Nayak, product manager at MicroStrategy.
“I feel like there’s always going to be a certain hesitancy… about whether your data is incomplete, if there’s missing data, if you’re using too many or too few variables,” Nayak said. “I feel like there’s always going to be a certain hesitancy around the data itself” also, he noted.
Meanwhile, “there’s another challenge that we’ve kind of seen here at MicroStrategy and that’s… getting those insights and those analytics to the masses,” Nayak went on to say.
Earlier, Roxane Edjlali, senior director of solution management at MicroStrategy, said it’s estimated that only about 60% of organisations are using AI and ML now as part of their data and analytics programs.
It was only as low as about 30% just a couple of years ago, Nayak said, noting: “We want to make sure that we’re reaching the rest of the organisation.”
And the “last thing that we’ve come across is now that you see these analytics, now you see these insights, now what?” Nayak asked rhetorically. “There’s still a tendency or a hesitancy about what people can do about it. Do they talk and collaborate about it? Do they develop business insights from it? Or do they just look at it and say, ‘oh, that looks nice’ and then forget about it? We need to make sure that the insights that we derive are relevant and meaningful.”
Other Challenges
Pointing to additional challenges, Tong said: “There’s so many problems I think we haven’t solved and I think one of the top ones is skill gap, which is still a challenge. When you look at analyst reports… about barriers to AI adoption, you can still see that skill gap is among the top challenges.”
There has been “this chasm which is preventing organisations from evolving from a basic form of analytics to an advanced form of analytics where they’re leveraging state-of-the-art machine learning models to deliver predictive insights,” according to Tong.
There are, meanwhile, many organisations that “struggle with acquiring scarce resources like data scientists or they just can’t acquire enough of data scientists and deliver results and solutions at the pace the business desires,” Tong said. “And it’s not just data scientists,” he said, pointing to the challenges of “responsibly deploying machine learning workloads.”
Data scientists also need to “collaborate with other experts in DevOps and data engineering in the business,” Tong said, adding: “It’s a complex process today and a lot of experts are required…. This operational challenge ties back to what’s built around trust. So, at the end of the day, all these processes need to come together so you build these models that might be opaque but you need to have the consumer kind of convinced that they trust these predictions. You may have a great customer churn model but they want to understand why does this model claim that this customer is at risk of churn. And those providing that trust, those insights, I think, are still challenges in the organisation.”
But Tong has seen many improvements in the industry, including through AWS and through “simplifying the automation of workflows from prototype to production,” he went on to say.
For example, “I’ve seen improvements around AutoML so that it lowers the bar so that non-experts can also build production-ready models as well as improvements around responsible AI tools that deliver explainable insights into models,” he added.